Foundational Research
Risk Communication
Increasing our understanding of attitudes and perceptions of AI to advance the development of trustworthy AI for improved environmental decision making.
Trust and Trustworthiness
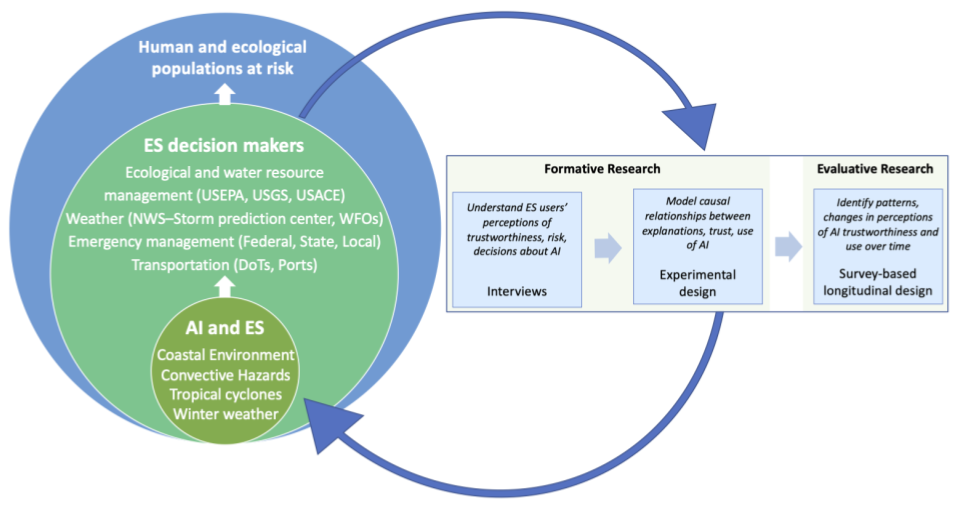
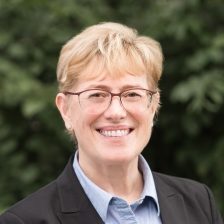
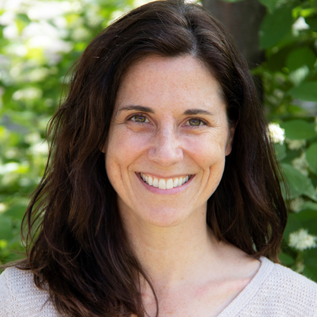
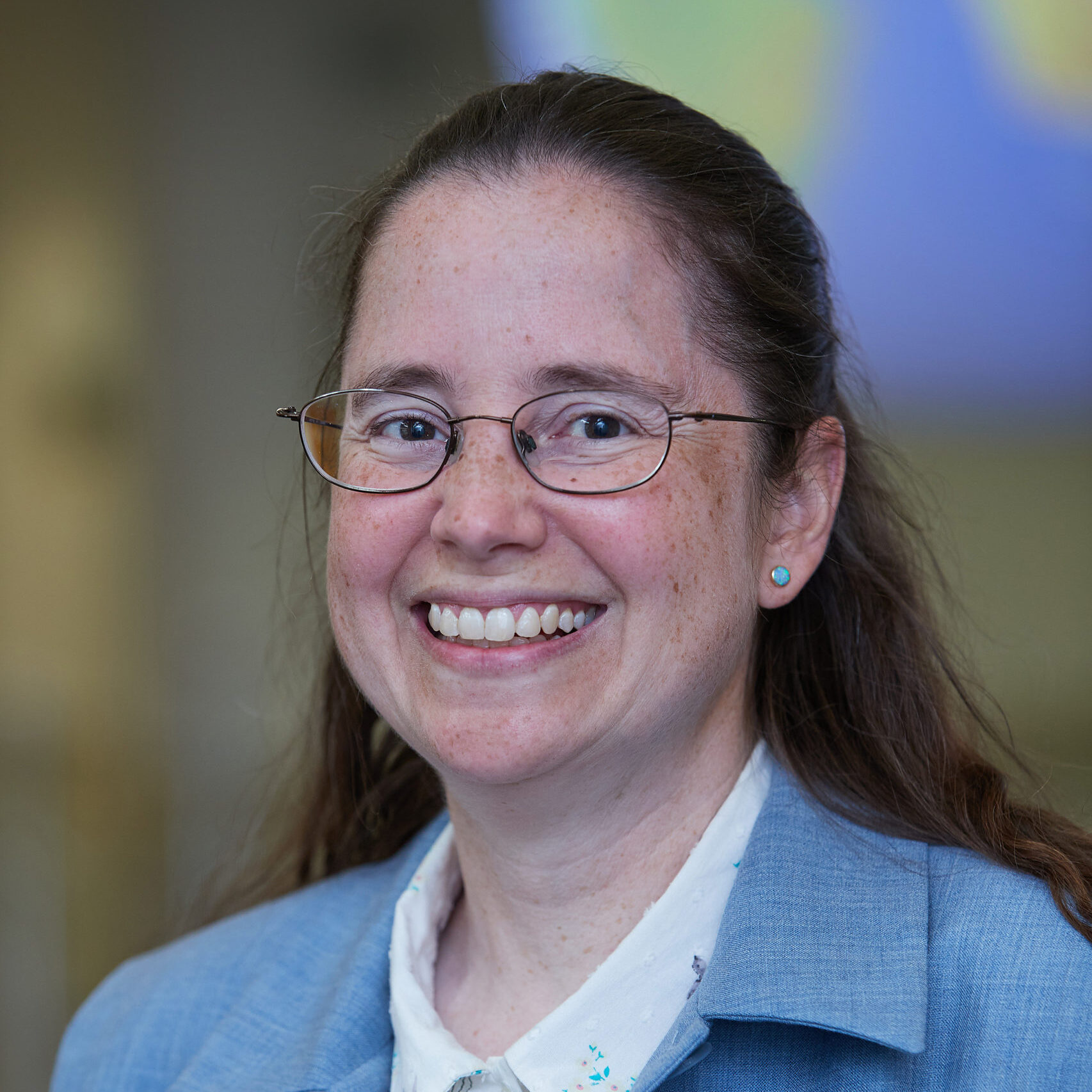
“When [weather forecasters] cannot easily understand the workings of a probabilistic product or evaluate its accuracy, this reduces their trust in information and their willingness to use it.”
From Recommendations for Developing Useful and Usable Convection-Allowing Model Ensemble Information for NWS Forecasters (Demuth et al. 2020)
Our risk communication team work on furthering our understandings of trust and trustworthiness in the context of AI and Earth system science. Our team’s goals include:
- Increase knowledge and understanding of how transparency, explanation, reproducibility, and representation of uncertainty influence trust in AI for environmental science (ES) for influential user groups.
- Develop models to estimate how attitudes and perception of AI trustworthiness influence risk perception and use of AI for ES
- Develop principled methods of using this knowledge and modeling to inform development of trustworthy AI approaches and content, and the provision of AI-based information to user groups for improved environmental decision making.
Highlighted Publications and Releases
Wirz, C. D., Demuth, J. L., Bostrom, A., Cains, M. G., Ebert-Uphoff, I., Gagne II, D. J., Schumacher, A., McGovern, A. & Madlambayan, D. (2025). (Re) Conceptualizing trustworthy AI: A foundation for change. Artificial Intelligence, 104309. https://www.sciencedirect.com/science/article/pii/S0004370225000281
McGovern, A., Demuth, J., Bostrom, A., Wirz, C. D., Tissot, P. E., Cains, M. G., & Musgrave, K. D. (2024). The value of convergence research for developing trustworthy AI for weather, climate, and ocean hazards. npj Natural Hazards, 1(1), 13.https://doi.org/10.1038/s44304-024-00014-x
Bostrom, A., Demuth, J.L., Wirz, C.D., Cains, M.G., Schumacher, A., Madlambayan, D., Bansal, A.S., Bearth, A., Chase, R., Crosman, K.M. and Ebert‐Uphoff, I., (2024). Trust and trustworthy artificial intelligence: A research agenda for AI in the environmental sciences. Risk Analysis, 44(6), 1498-1513. https://onlinelibrary.wiley.com/doi/10.1111/risa.14245
Severe Convection
Trustworthy AI for Severe Convection with User Interviews
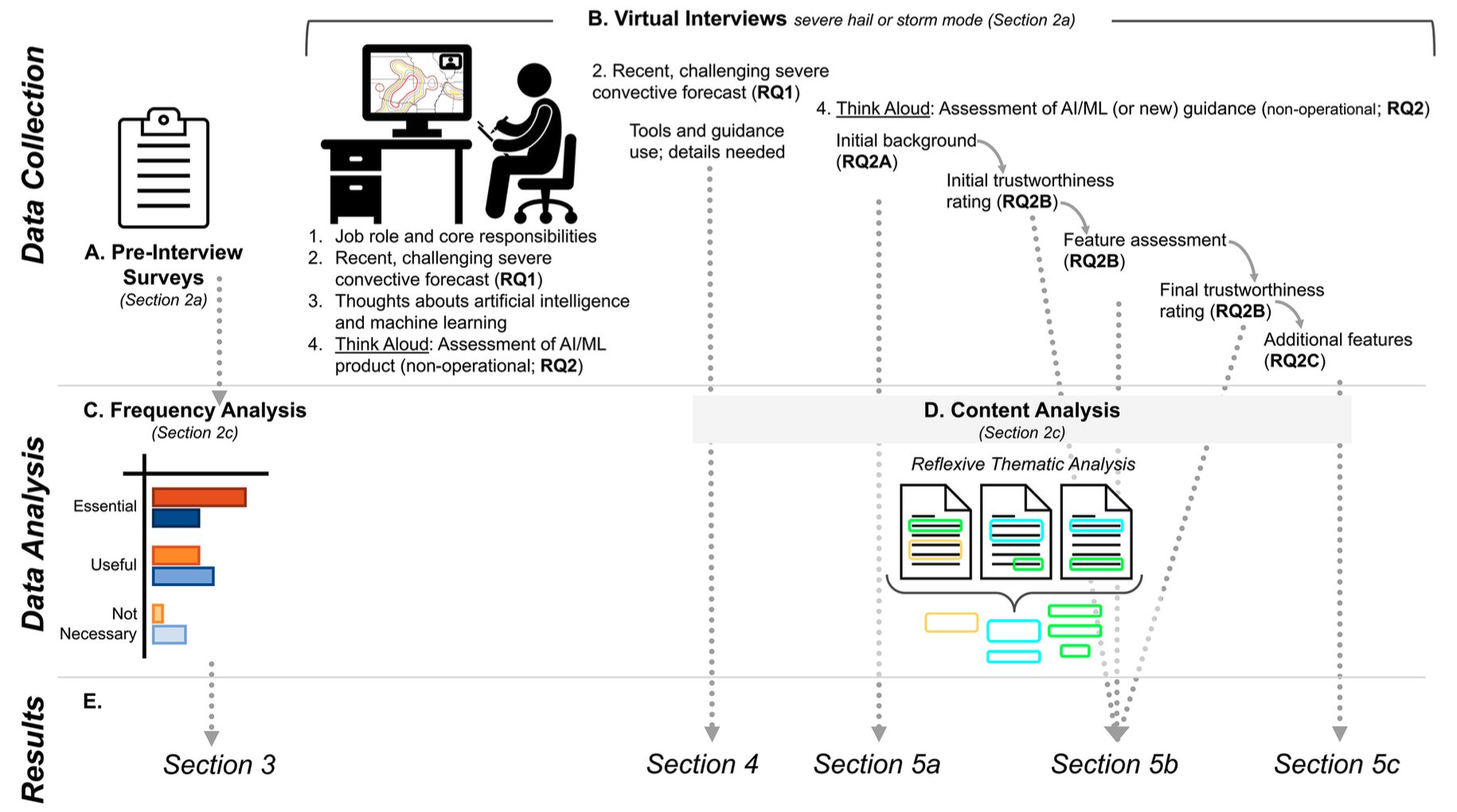
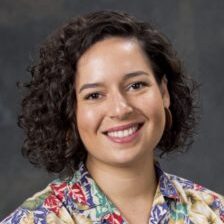
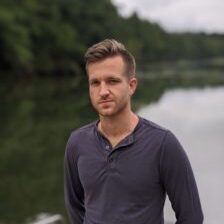
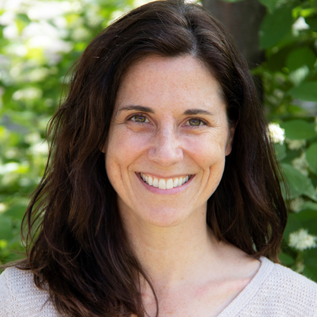
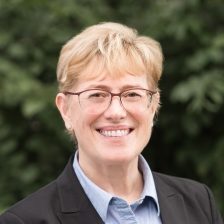
Building trust in AI-driven weather guidance is essential for integrating advanced machine learning (ML) models into operational forecasting. This project investigates how weather forecasters perceive the trustworthiness, explainability, and usability of AI-based predictions for severe convection. Through structured interviews with National Weather Service (NWS) forecasters, we aim to: (a) identify unmet informational needs to guide future AI/ML development, (b) assess perceptions of AI trustworthiness and interpretability, and (c) evaluate forecasters’ willingness to use a random forest hail prediction model and a convolutional neural network (CNN) storm mode prediction system. Our structured interview protocol is designed to be adaptable across public and private sectors and multiple use cases.
Key achievements include using a mixed-methods quantitative and qualitative approach to understand how National Weather Service (NWS) forecasters 1) make guidance use decisions within their operational forecasting process and 2) assess the trustworthiness of prototype guidance developed using artificial intelligence (AI). When taken as a whole, our findings illustrate that forecasters’ assessment of AI guidance trustworthiness is a process that occurs over time rather than automatically and suggest that developers must center the end user when creating new AI guidance tools to ensure that the developed tools are useful and used.
Related Projects: Convective Initiation and Hail Nowcasting
Highlighted Publications and Releases
M. G. Cains, C. D. Wirz, J. L. Demuth, A. Bostrom, D. J. Gagne, A. McGovern, R. A. Sobash, and D. Madlambayan. Exploring NWS forecasters’ assessment of AI guidance trustworthiness. Weather and Forecasting, 39(8):1219 – 1241, 2024. doi:10.1175/WAF-D-23-0180.1. https://doi.org/10.1175/WAF-D-23-0180.1
C. D. Wirz, J. L. Demuth, M. G. Cains, M. White, J. Radford, and A. Bostrom. National weather service (NWS) forecasters’ perceptions of AI/ML and its use in operational forecasting. Bulletin of the American Meteorological Society, 105(11):E2194–E2215, 2024. https://doi.org/10.1175/BAMS-D-24-0044.1
Winter Weather
Leveraging Social Science for Reproducible Supervised ML Hand Labeling
To improve the reproducibility and transparency of supervised machine learning (ML) training data, our team has integrated quantitative content analysis (QCA) from social science into the hand-labeling for machine learning applications process. We applied and refined this approach through two winter weather projects: the New York State Department of Transportation road surface conditions model and the New York Mesonet nighttime precipitation detection model. This work addresses challenges in ensuring consistent labels across diverse datasets while facilitating interdisciplinary collaboration. Through this work, our team established a scalable, interdisciplinary framework for improving the quality and reproducibility of ML training datasets, enhancing their value for Earth system science applications.
Related Projects: Winter Road Weather/Decision Making, Precipitation Detection from Cameras
Highlighted Publications and Releases
C. Wirz, C. Sutter, J. L. Demuth, K. J. Mayer, W. E. Chapman, M. G. Cains, J. Radford, V. Przybylo, A. Evans, T. Martin, L. C. Gaudet, K. Sulia, A. Bostrom, D. J. Gagne, N. Bassill, A. Schumacher, and C. Thorncroft. Increasing the reproducibility, replicability, and evaluability of supervised AI/ML in the Earth systems science by leveraging social science methods. Earth and Space Science, 11(7):1–17, 2024. https://doi.org/10.1029/2023EA003364
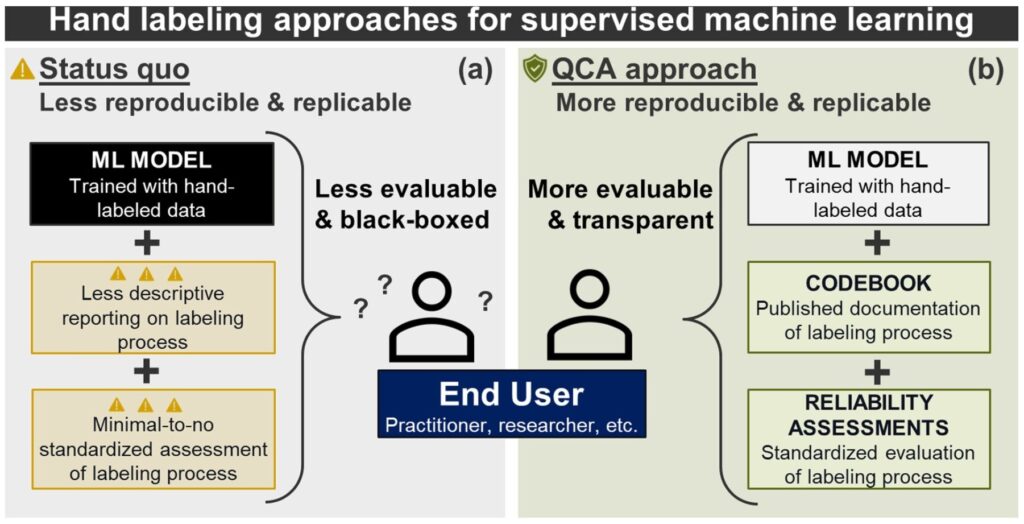
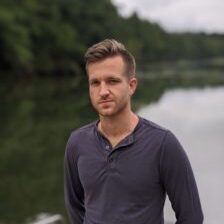
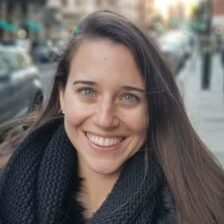
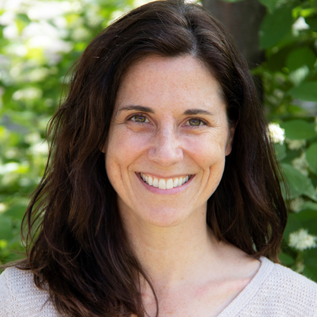
Trustworthy AI for Winter Precipitation Type with Users
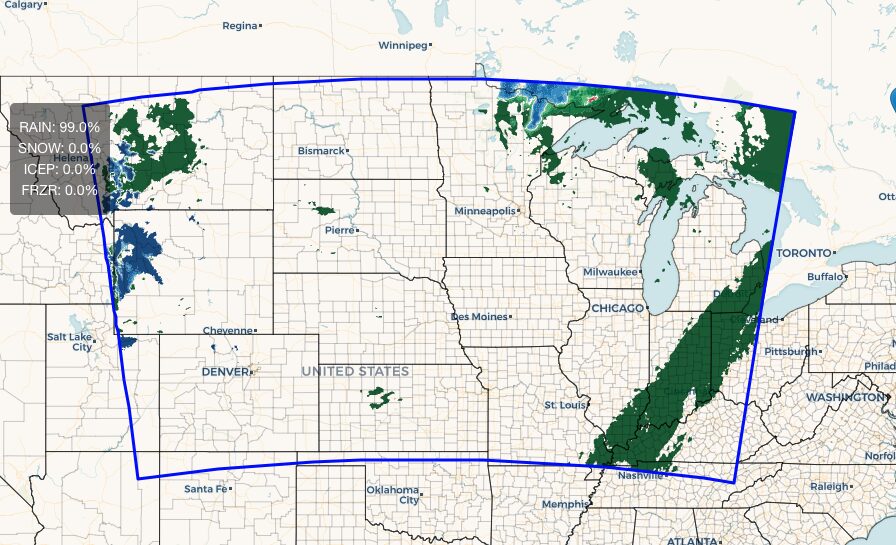
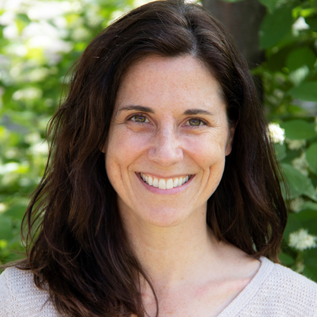
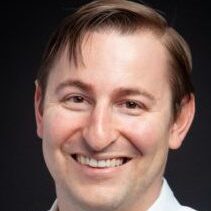
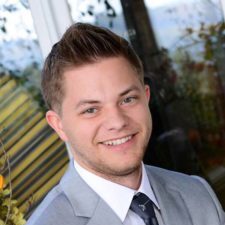
This project focuses on co-developing a machine learning (ML) tool for winter precipitation-type (p-type) nowcasting and prediction that is both trustworthy and usable by weather forecasters, transportation officials, and other partners. The team employs a mixed-methods approach—combining interviews and naturalistic observations—to understand users’ forecasting processes, informational needs, and decision-making related to p-type, as well as their perceptions of the trustworthiness and interpretability of the ML model.
Significant achievements include the development of interactive tools and model outputs, such as ensemble and timing predictions, for evaluation with National Weather Service (NWS) forecasters. Our team has also created a structured interview protocol to assess forecasters’ interactions with the model, including how they interpret model uncertainty and performance over time and space. This work will help refine the model’s trustworthiness, transparency, and utility in operational forecasting.
Related Project: Winter Weather P-Type Analysis and Forecasts
Nearshore Coastal
Trustworthy XAI for FogNet with User Interviews
Our team is working to co-develop a think-aloud interview guide to help National Weather Service (NWS) forecasters provide feedback on and critically assess AI/ML coastal fog guidance. Building on earlier severe forecaster interviews, the team is expanding research to gather empirical data on forecasters’ perceptions and reactions to case studies and explainable AI (XAI) results. By conducting interviews with 13 NWS forecasters from the Eastern, Southern, Western, and Alaskan regions, the project examines key variables influencing expert decision-making in fog forecasting.
As of 2024, our team has completed stakeholder interviews, publishing interview materials and the AI/ML interview guide, and developing research questions and analysis plans for two empirical papers. These efforts are advancing our understanding of forecaster interactions with XAI models and how to improve fog forecasting guidance.
Related Project: Coastal Fog Predictions (FogNet)
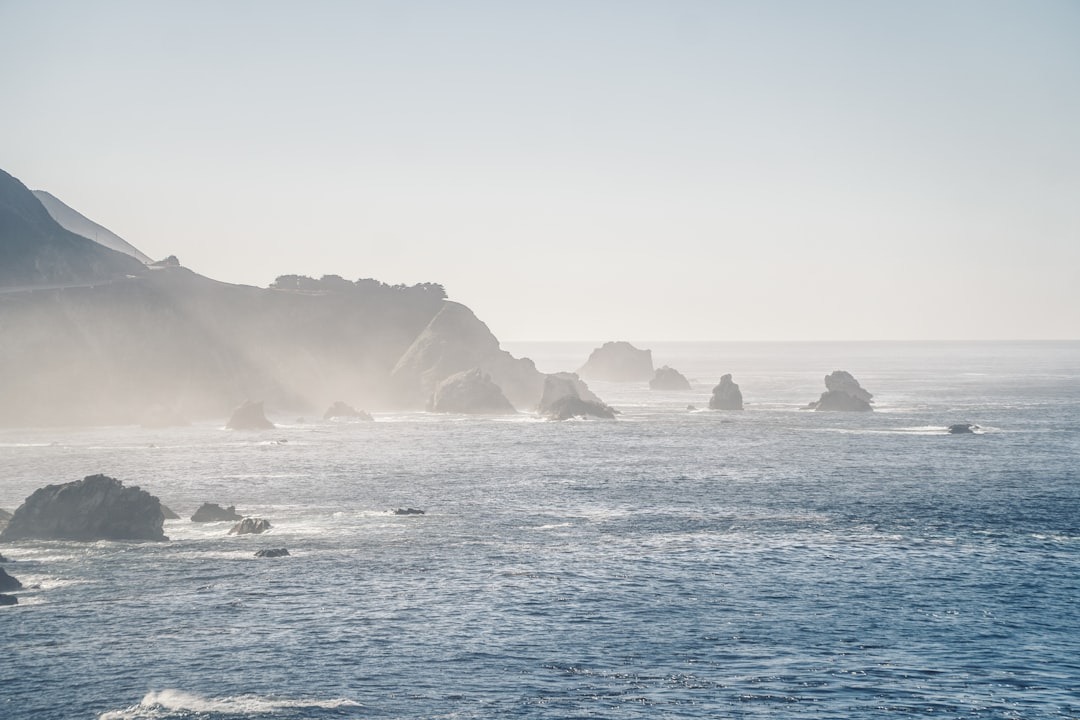
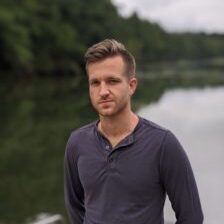
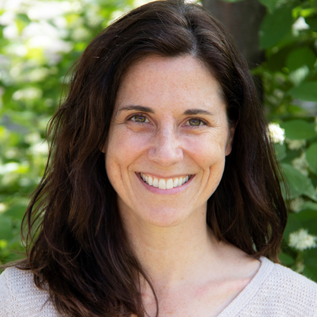
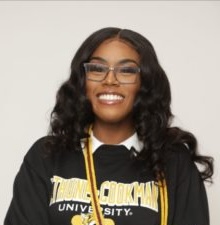
Highlighted Publications and Releases
C. D. Wirz, J. L. Demuth, M. G. Cains, M. White, J. Radford, and A. Bostrom. National weather service (NWS) forecasters’ perceptions of AI/ML and its use in operational forecasting. Bulletin of the American Meteorological Society, 105(11):E2194–E2215, 2024. https://doi.org/10.1175/BAMS-D-24-0044.1
Risk communication for Cold Water Temperature Prediction
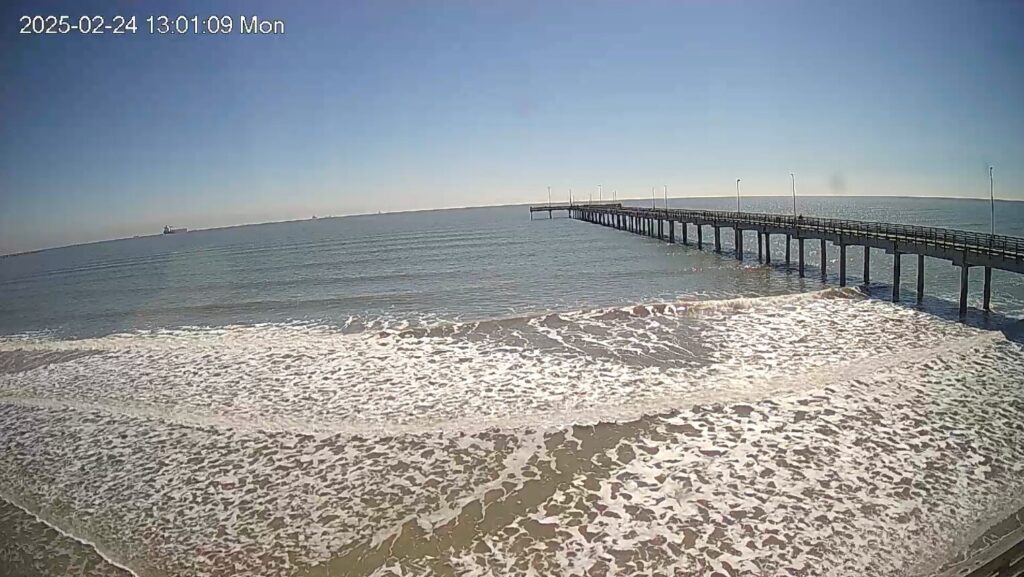
Highlighted Publications and Releases
Laguna Madre Water Temperature Prediction System for Mitigation of Cold-Water Events (Website)
The Risk Communication team has been heavily involved with our sea turtle conservation efforts. They have been advising the development of stakeholder interviews and mentoring Ph.D Candidate Miranda White to support our ongoing, convergent research efforts. This mentorship and training opportunity has enabled junior researchers to take leadership in partner engagement, having a direct impact on the Laguna Madre’s response to sea turtle cold stunning events. Please read more about our sea turtle conservation project, linked below.
Related Project: Sea Turtle Conservation
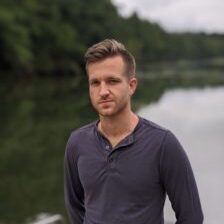
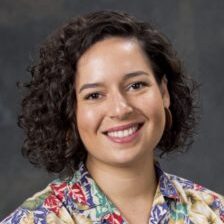
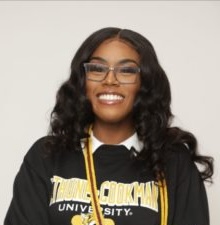
Oceans
Trustworthy AI for Ocean Eddy Prediction with Users
Our team is co-developing a think-aloud interview guide to identify factors influencing trust in AI/ML Loop Current Eddy (LC/LCE) prediction products among expert users, specifically metocean specialists from oil and gas companies operating in the Gulf of Mexico. Our work builds on insights from prior interviews with severe weather and coastal fog forecasters to assess which trust factors generalize across expert domains.
Related Project: Physics-Inspired AI for Offshore Oceanography
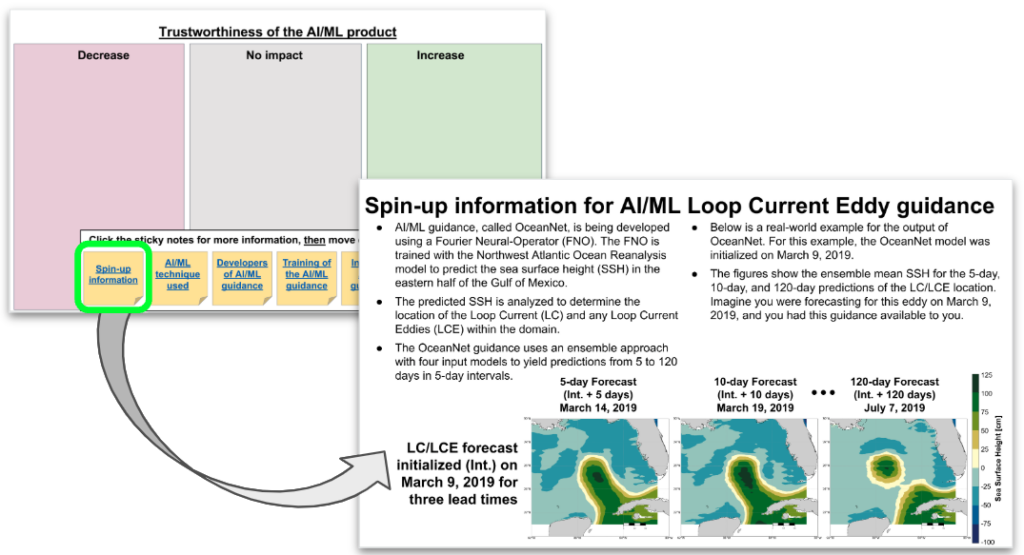
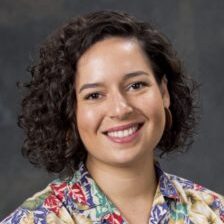
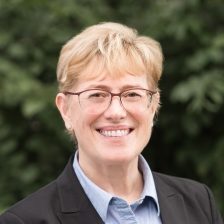
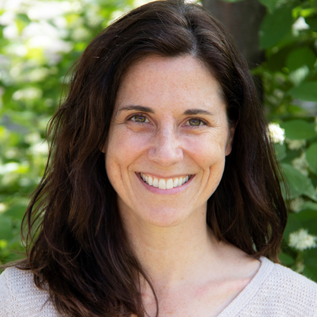
Advancing AI in Earth System Science Research
Join us to unlock AI’s potential in science.